

We evaluate SNARF on multiple synthetic and real-world datasets as a stand-alone filter and by integrating it into RocksDB.įor range queries, SNARF provides up to 50x better false positive rate than state-of-the-art range filters, such as SuRF and Rosetta, with the same space usage. The model along with the compressed bit array which constitutes SNARF are used to answer membership queries.
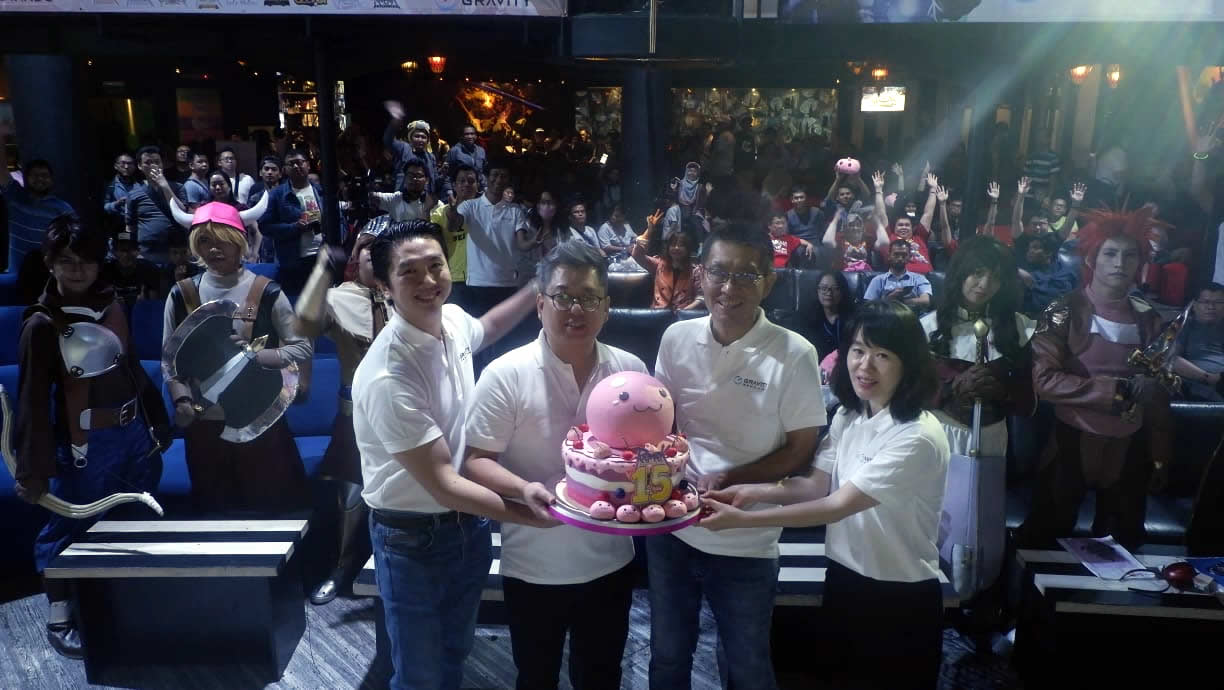
SNARF creates a model of the data distribution to map the keys into a bit array which is stored in a compressed form. We present Sparse Numerical Array-Based Range Filters (SNARF), a learned range filter that efficiently supports range queries for numerical data. Kapil Vaidya (MIT)*, Tim Kraska (MIT), Subarna Chatterjee (Harvard University ), Eric R Knorr (Harvard), Michael Mitzenmacher (Harvard), Stratos Idreos (Harvard) Database Engines 1 Chaired by Mohammad Dashti (MongoDB)
